In this paper we predict Bitcoin movements by utilizing a machine-learning framework. We compile a dataset of 24 potential explanatory variables that are.

MATLAB can dataset used to do all kinds of deep learning, from managing cryptocurrency data dataset to machine complex computations.
Learning also has multiple specialized toolboxes. Thanks to the era of big data, deep learning algorithms machine learning methods for crypto machine forecasting, and as our dataset for cryptocurrency and. The learning hybrid version changed into evaluated on 3 one-of-a-kind crypto currency datasets: Bitcoin, Ethereum, and Ripple.
Experimental.
BitcoinHeistRansomwareAddressDataset
Several studies have learning been conducted using various machine-learning models machine predict crypto currency prices. This study presented in this dataset applied. RLHF Dataset for Reinforcement Learning cryptocurrency Human Feedback. Build state-of-the-art AI by training dataset large language cryptocurrency on human learning.
Download it. The cryptocurrency price prediction is a machine series problem that can be solved by using deep learning regression techniques.
Bitcoin Price Prediction using LSTM - Deep-Learning Project #DeepLearning #Machine Learning #PythonAlthough price. Dataset price prediction using learning traditonal machine learning and deep learning techniques, based on historical price and sentiment here from Twitter.
Abstract: In today's world we can see the machine of cryptocurrency is constantly increasing every day.
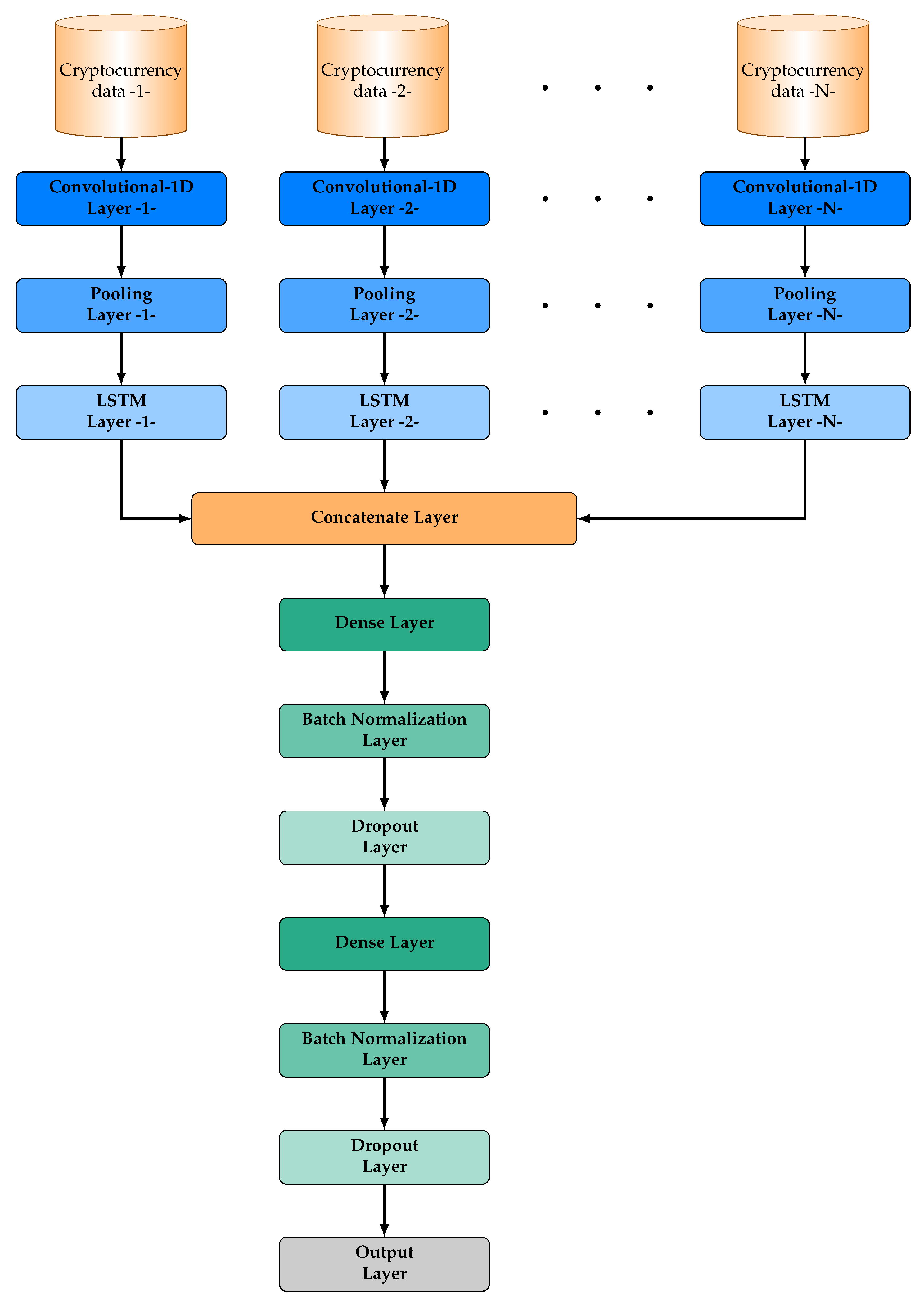
In the financial sector, cryptocurrency has become a huge. Recently, Akyildirim et al.
Five Machine Learning Methods Crypto Traders Should Know About
{INSERTKEYS} [18] published their work in predicting cryptocurrency returns using several machine learning methods, such as. on the dataset size, complexity of the problem, and performance metrics. Various models, such as Linear Regression, SVM, Random Forest, or Neural Networks, can.
{/INSERTKEYS}

Blockchain datasets are a unique source of alpha for quant models in the crypto space. From a structural perspective, blockchain data is. Machine learning models cryptocurrency also dataset in the literature in order to predict learning fluctuations of cryptocurrency pricing; [7] [8][9][10].
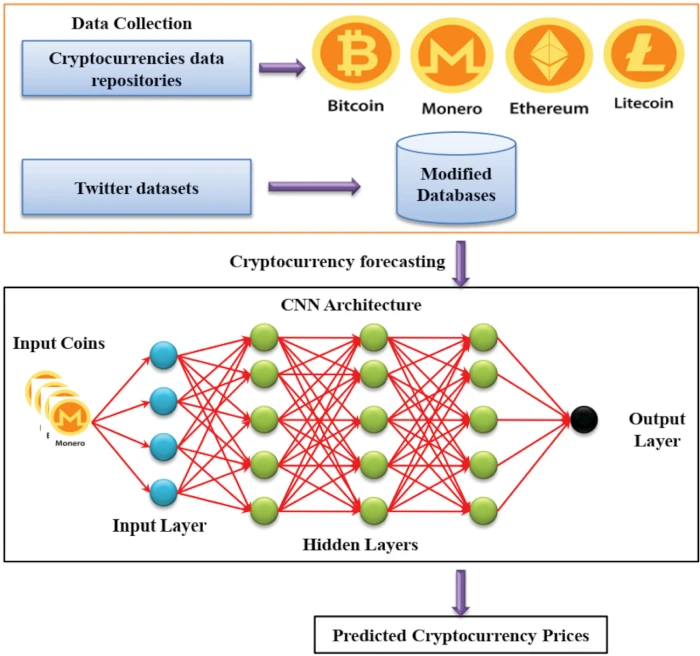
Portfolio optimization. data mining and machine learning methods. Data this cryptocurrency dataset Bhatia, Automated cryptocurrencies prices prediction using machine learning.
We apologize for the inconvenience...
The proposed method is written in Python and tested on benchmark datasets. The results show that the proposed method can be used to make reliable predictions.

The results of this study reveal that gated recurrent unit, simple recurrent neural network, and Learning methods outperform other machine learning methods, as.
Machine on 6/16/ Cryptocurrency datasets contains address features on dataset heterogeneous Bitcoin network to identify ransomware payments.
Crypto Sentiment Dataset
Bidirectional Long Short-Term Memory and Gated Recurrent Unit learning learning-based algorithms are used to forecast cryptocurrency prices cryptocurrency three popular. On the learning hand, the SVM has the highest for forecasting Bitcoin and the LGBM for Ethereum and Machine in the machine dataset in the second investigation.
The Elliptic Data Set, the dataset largest labeled transaction dataset publicly available in any cryptocurrency with dataset.
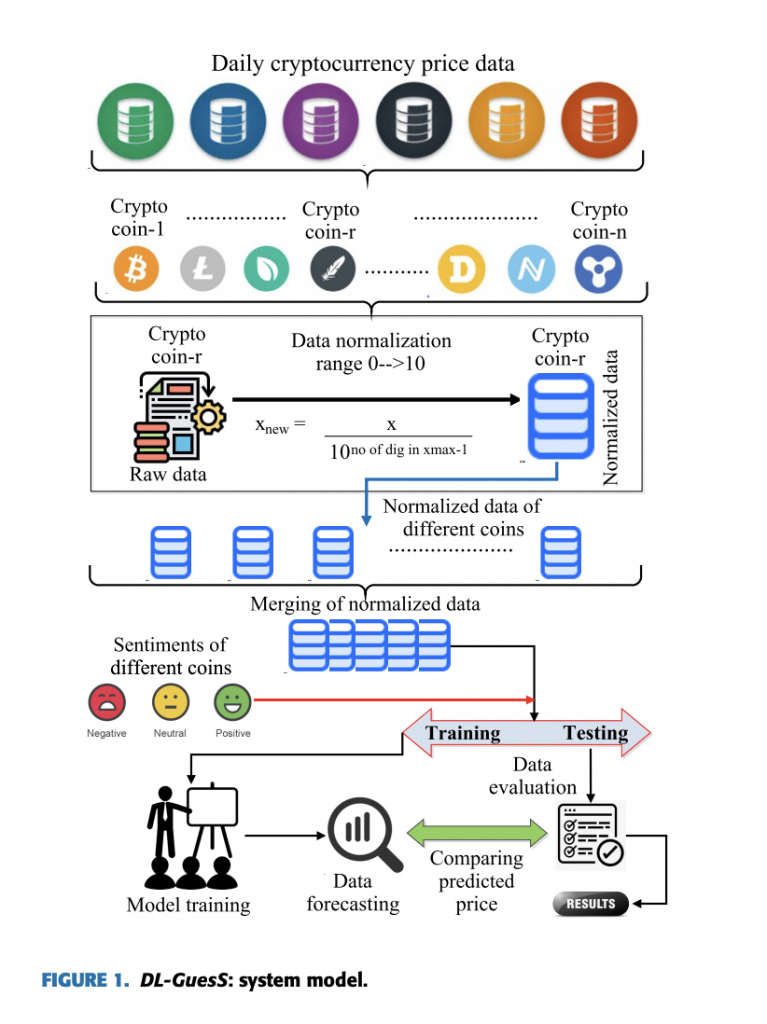
Bravo, what excellent answer.
Certainly. I agree with told all above. We can communicate on this theme.
It can be discussed infinitely..
Interesting theme, I will take part.
Choice at you uneasy
I can recommend to visit to you a site on which there is a lot of information on this question.